How Does Retrieval-Augmented Generation (RAG) Boost AI Performance?
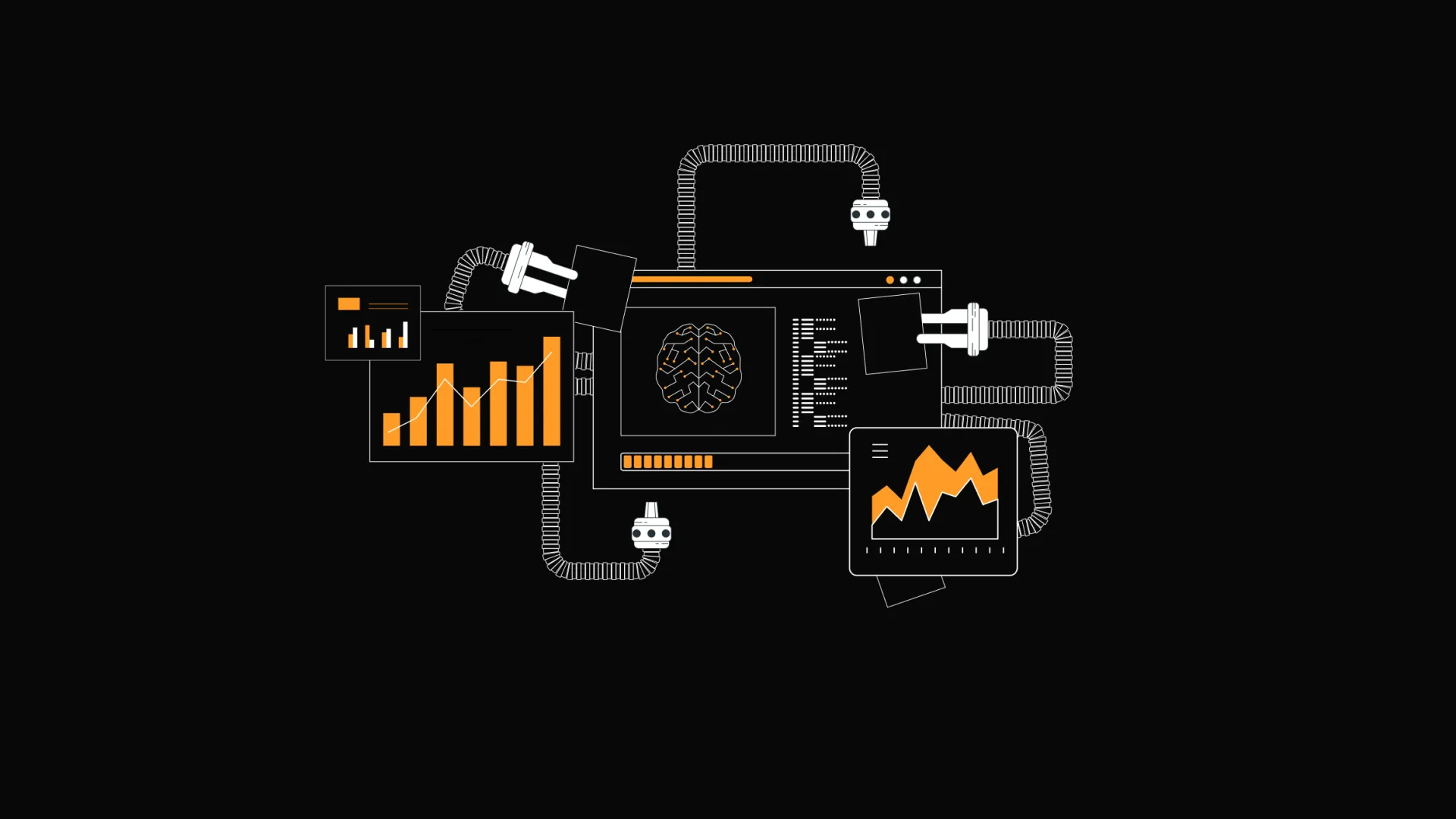
In AI, the constant push for more accurate, context-aware, and insightful responses has led to the development of multiple techniques that offer ways to enhance the knowledge base of LLM-based solutions. One such technique is Retrieval-Augmented Generation (RAG), a method that integrates the strengths of information retrieval with the capabilities of generative models.
Understanding Retrieval-Augmented Generation
Retrieval-augmented generation combines generative models with a retrieval mechanism. Traditional generative models rely solely on their training data, which, despite being extensive, has limitations in providing up-to-date or precise information. RAG addresses this limitation by incorporating a retrieval step that fetches relevant information from relevant data sources before generating a response.
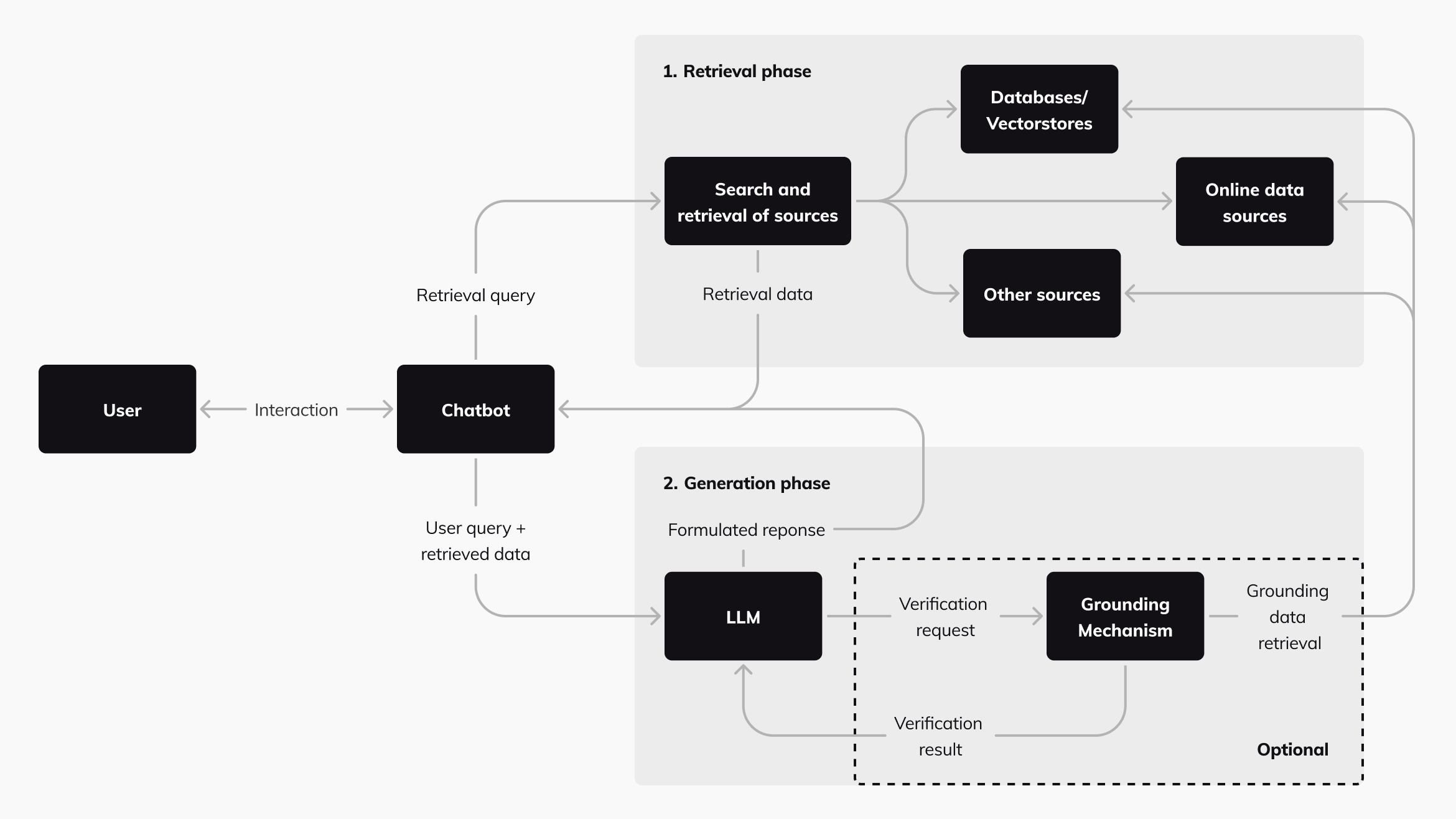
The Mechanism of RAG
The RAG framework consists of two main stages:
1. Retrieval Phase:
A retrieval query can be a simple raw input from the user (question or task) or an agent-refined query that captures the objective of the interaction with the user.
Search and retrieval is a mechanism that can access various data sources where it can search and retrieve relevant data from.
Data sources can be databases, vector stores, online data sources, APIs, files, conversations, e-mails or any other trusted source that contains up-to-date and relevant data on the topic.
2. Generation Phase:
The retrieved information, along with the original query, is then provided to the generative model where the response is generated.
Optional: The system can be further enhanced by a grounding mechanism that verifies the generated text's factuality, thus providing a means of self-verification and another layer to combat model hallucinations. The grounding mechanism fixes the issues by comparing entities (names, dates, locations, figures, etc.) from the generated response with the source data.
Advantages of RAG
Improved Accuracy
By integrating real-time information, RAG enhances the accuracy of AI responses. This is particularly beneficial for applications requiring current knowledge, such as customer support, news generation, and dynamic content creation.
Contextual Relevance
RAG ensures that generated responses are more contextually relevant by incorporating specific details from the retrieved data. This results in more coherent and context-aware outputs, enhancing user experience.
Versatility
RAG can be scaled to work with various types of databases and knowledge sources, making it adaptable across different domains and industries. Whether it involves legal documents, medical literature, or technical manuals, RAG can tailor its responses accordingly.
Reduction in Errors
Generative models sometimes produce plausible-sounding but incorrect or nonsensical answers, known as "hallucinations." By grounding the generation process in retrieved information, RAG reduces the likelihood of such errors.
Cost-effectiveness
The use of RAG replaces the need to frequently fine-tune generative models with the most recent data. This saves the client money, time, and resources.
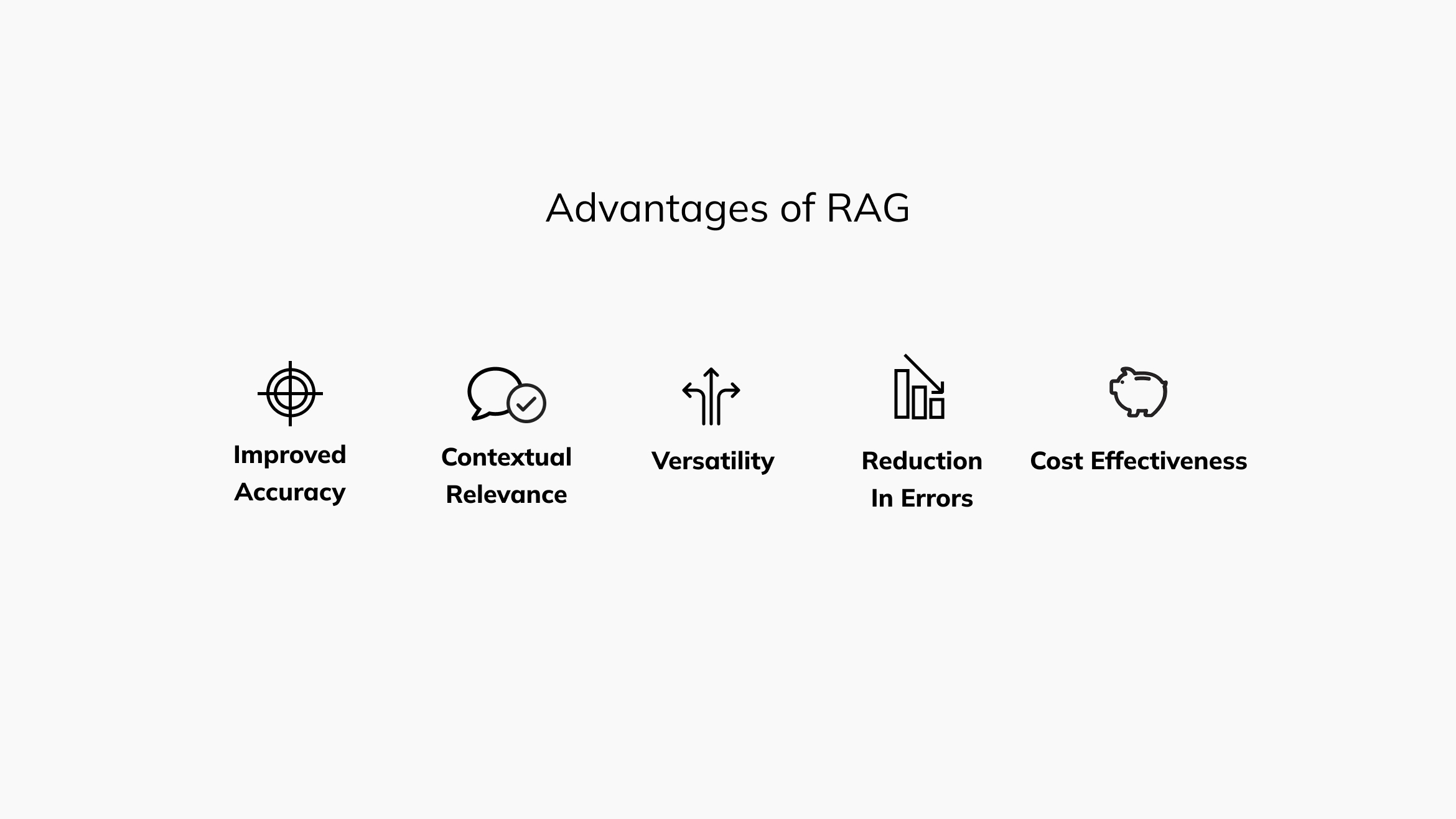
Applications of RAG
The versatility of Retrieval-Augmented Generation makes it applicable across a wide range of industries and use cases:
Customer Support:
RAG can enhance chatbot performance by providing accurate and contextually relevant responses, improving customer satisfaction, and reducing resolution times.
Content Creation:
In journalism and content marketing, RAG can assist in generating articles that are both informative and engaging, drawing from a vast pool of real-time data.
Healthcare:
Medical professionals can leverage RAG for accurate and current information retrieval, aiding in diagnostics and treatment plans.
Legal Research:
Lawyers and legal researchers can use RAG to sift through extensive databases of case law and legal documents, ensuring access to the most pertinent information.
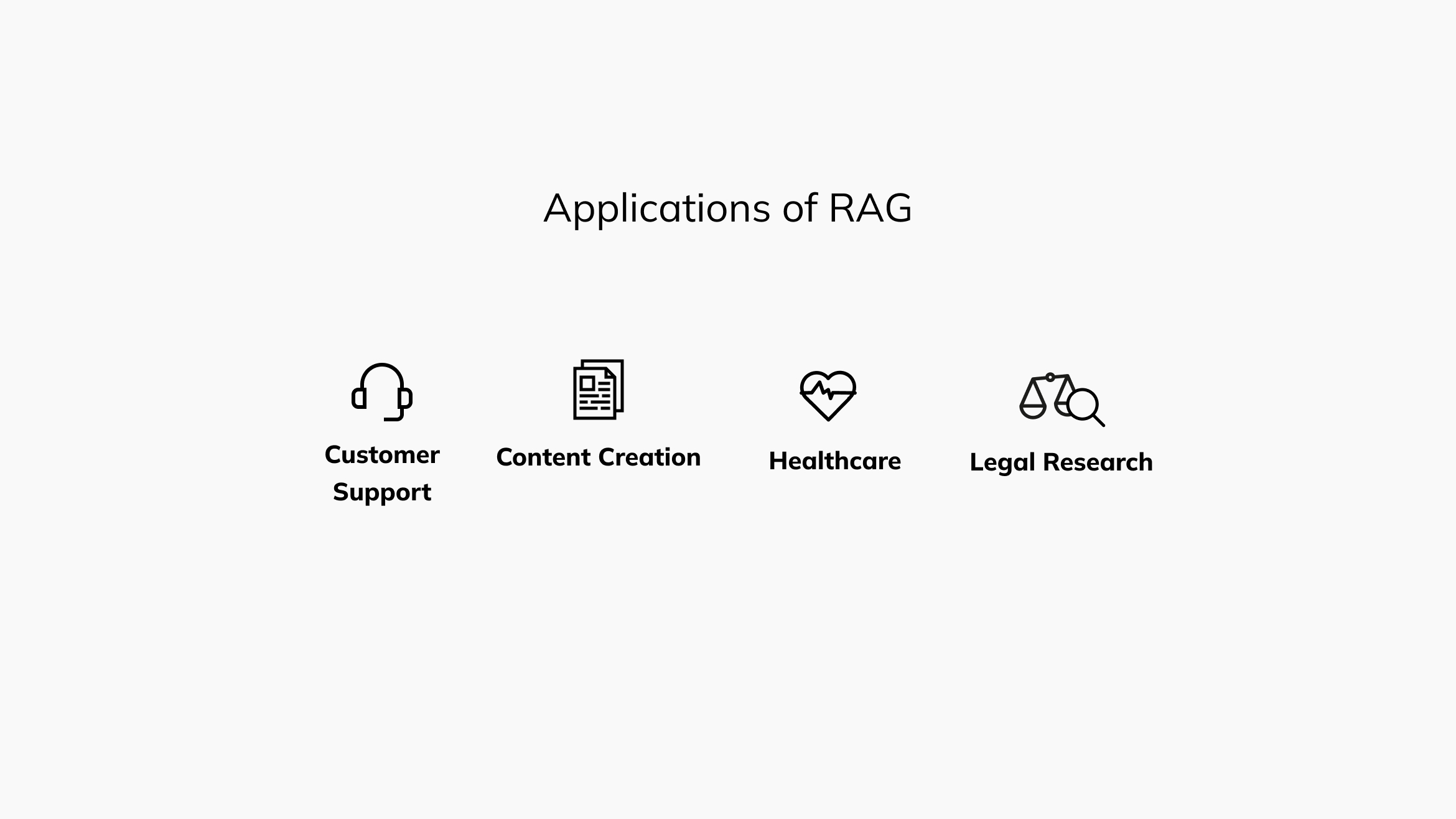
Challenges and Future Directions
While RAG represents a significant advancement in AI technology, it is not without challenges. Ensuring the quality and relevance of retrieved information is critical, as is managing the balance between the computational/infrastructural overhead associated with the retrieval process compared to generative model fine-tuning. Additionally, privacy and security concerns must be addressed, especially when dealing with sensitive information.
Future developments in RAG may focus on improving retrieval algorithms, enhancing the integration between retrieval and generation phases, and expanding the range of accessible knowledge bases. As AI continues to evolve, the synergy between retrieval and generation promises to unlock new levels of intelligence and capability.
Conclusion
Retrieval-augmented generation stands at the forefront of AI innovation, merging the potential of generative models with the precision of information retrieval. By bridging the gap between static knowledge and dynamic information, RAG offers a powerful tool for creating more accurate, relevant, and contextually aware AI applications. As this technology continues to mature, its impact will be significant across numerous domains, advancing the capabilities of AI.